Datafi Resources
Thank you! Your submission has been received!
Oops! Something went wrong while submitting the form.
Sorry, we couldn't find any results matching your search.
Don't give up! Check the spelling or rephrase your search query.
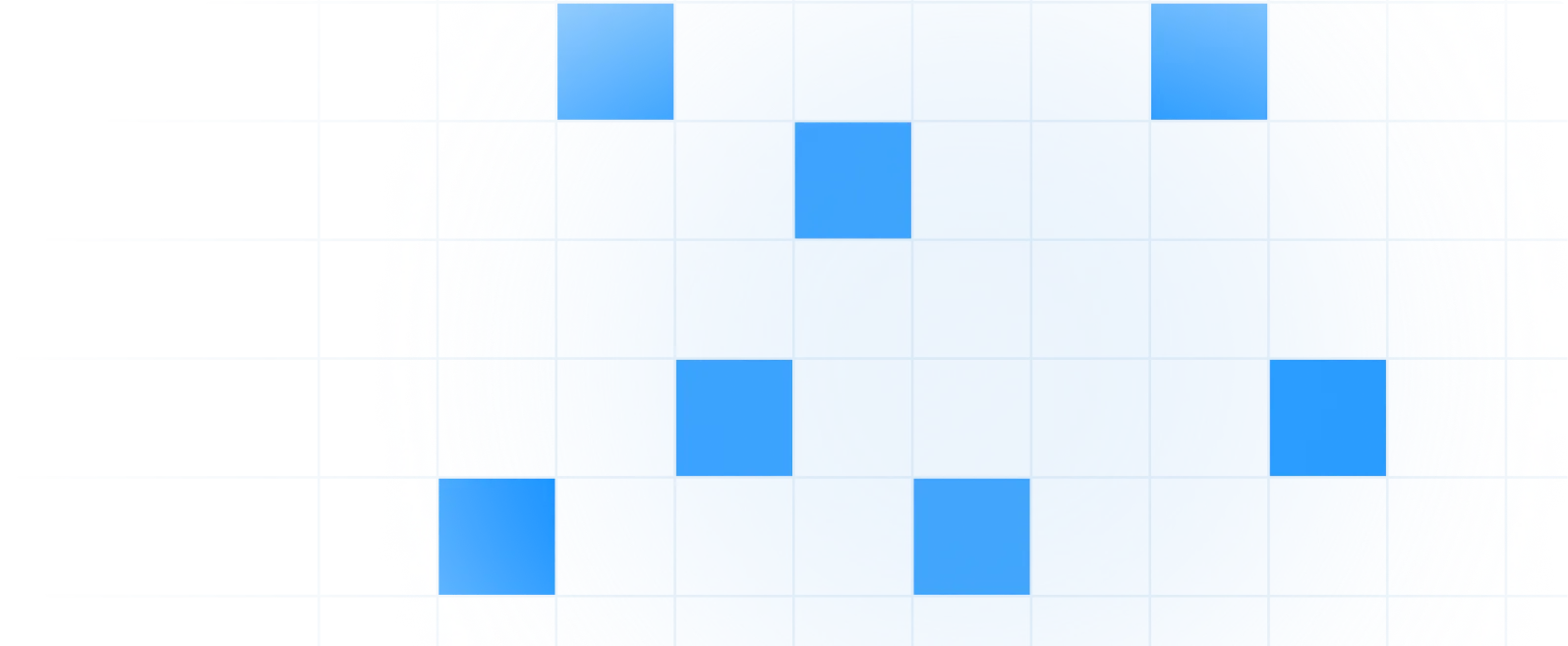
Get started with Datafi today
Datafi is an intelligent agent for work. Making data accessible and meaningful for everyone, anywhere.
Thank you! Your submission has been received!
Oops! Something went wrong while submitting the form.